A non-stop flow of website click stats, customer transactions, user feedback or reports can sometimes make you feel a bit crazy.
What a challenge it is to find insights from all this big data when it just keeps pouring in!
It’s no wonder that many company owners are turning to AI. AI technologies have made their way into almost every industry, and they’re proving to be reliable at analyzing massive datasets.
This blog post focuses on how AI can help with big data analysis, its benefits, and tricky nuances that may appear while trusting artificial intelligence.
And if you need personalized advice on implementing AI in big data in your specific field, we’re open to conversation. Drop us a line, and our AI developers with experience across 10+ industries will help you find the best way to apply AI to your project.
Contents
- What’s Big Data and How It’s Processed?
- How Can AI Help Analyze Big Data?
- Pros Of Using AI for Big Data Analysis
- Use Cases of AI in Data Analysis Across Industries
- Nuances You Need to Know before Adopting AI for Big Data
- Final Thoughts
What’s Big Data and How It’s Processed?
If your business is tied to digital processes, you have to deal with big data every day. Whether you’re running an online shop, managing a healthcare app, or working in finance, big data is always there in your daily workflow.
The goal of big data is to provide useful information about users, such as their shopping behavior, inquiries, transactions, and overall engagement. These valuable insights help understand how to improve the level of services or streamline business processes. However, the key information is often buried in a flood of unstructured, unnecessary data. To extract the needed insights, big data must be carefully processed.
You can do it in two ways—by using traditional methods of data analysis or implementing artificial intelligence into your software. Let’s take a look at the traditional methods first and how they work.
Traditional Methods of Big Data Analysis
Method | How it works | Limitations |
Data Warehousing | Helps organize and keep structured data (texts, numbers) from various resources in one storage system. | Good for structured data but not suitable for unstructured or fast-growing datasets. |
Statistical Analysis | Uses math-based methods to discover trends, connections, or patterns in data. | Works well with only clean and organized data, needs a lot of manual work. |
Query-Based Analysis | Uses commands in SQL (Structured Query Language) to interact with databases. | Struggles with unstructured or real-time data. |
Visualization Tools | Transforms data into visual formats like charts, graphs, or dashboards. | Relies on good-quality data and can have trouble managing very large or complex data sets. |
ETL Processes | Takes data from different sources, fixes it up, and stores it in a database. | Can be slow and have trouble handling the size or speed of modern big data |
Although traditional methods have been around for years, they all have one common limitation—working in real-time. On top of that, they can’t keep up with all the types of data at its speed.
Traditional methods usually need a lot of manual work to handle raw data, so you need to clean and organize data manually before passing it to the program to analyze. This takes too much time and leads to missed chances and opportunities.
AI, though, can do all that work much faster, uncovering the needed insights on the spot.
Let’s take a closer look at how AI makes big data analytics easier and more efficient.
How Can AI Help Analyze Big Data?
Finding useful insights in raw data is like looking for a needle in a haystack. Found everything you need today? Good for you! But guess what—tomorrow there’ll be more information to sort out. It’s a never-ending cycle.
With artificial intelligence, analyzing and interpreting sense out of vast volumes of data becomes lightning-fast. How does AI make it?
AI is more than just organizing data; it gets trained, predicts and adapts to changing information. AI-analyzing algorithms are based on different techniques. Each of them tackles specific tasks in data analysis, giving results unbelievably fast. They are:
- Machine learning
- Natural language processing
- Deep learning
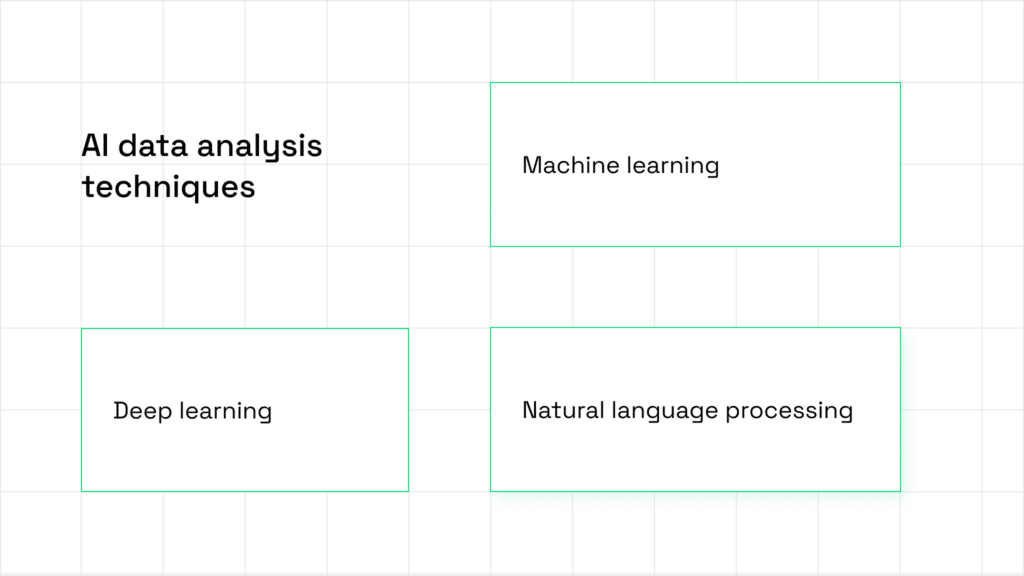
Machine learning
The ML mechanism helps artificial intelligence better ‘understand’ patterns, learn from them, combine, and predict new ones. This superpower allows AI to catch fraud, monitor risks, and adapt to changes.
Mastercard uses artificial intelligence in their software to spot payment fraud. The smart system monitors transactions as they are made and detects unusual actions that may be fraudulent. At the same time, AI learns and adapts to new fraud schemes, making payments safer for everyone.
Natural Language Processing
NLP is the way AI catches the human requests, analyzes them, and gives responses. So, artificial intelligence can easily process emails, customer queries, and other types of text content.
With AI-driven tools, Coca-Cola studies how their customers behave on social media. This helps them understand their audience and create better marketing plans.
Deep Learning
DL functions similarly to the human brain. It uses multilayered artificial neural networks, which allow AI to comprehend videos, audio, photos, and even read medical scans such as MRIs. With DL, AI can recognize and remember patterns, making it ideal for offering similar content to users on social media or uncovering undesirable content.
Artificial intelligence helps Facebook moderate content. The AI-based algorithms detect photos and videos with nudity or violence and either block or delete them.
The combination of these AI techniques allows you to take a huge step forward in optimizing your business processes and take sharper and more confident decisions.
Pros of Using AI for Big Data Analysis
If you’ve ever used AI for personal purposes, you’ve probably noticed how it has changed the way we work with information. For example, now it’s possible to upload a long document and ask ChatGPT to make its summary instead of reading all 20 pages. It pretty much saves time, doesn’t it?
However, AI is not only about making life easier in personal use. In business, artificial intelligence has already proven its brilliance in many ways, including writing all types of text content, creating photo and video materials, communicating with customers, and, most importantly, analyzing large volumes of big data.
Let’s look into the key benefits of implementing AI for big data analysis in business.
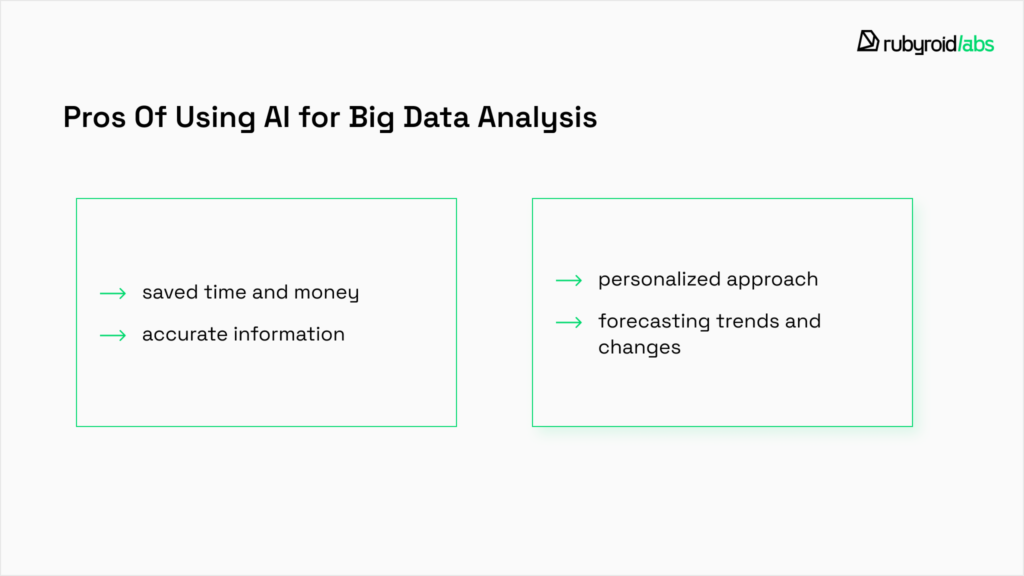
- Saved time and money. While others are busy analyzing and cleaning data manually, AI delivers key insights that you can implement on the go. It monitors and analyzes data in real-time, saving your time, cutting costs, and making better use of your team.
- Accurate information. AI’s innovative algorithms can search, analyze, group, and deliver accurate information with a very low chance of errors. This is especially critical for industries like finance, healthcare, and manufacturing, where mistakes can be highly costly.
Reliable insights are also valuable in marketing analytics platforms where an endless flow of statistics needs order and key data points to enhance marketing strategies. - Client-focused approach. Artificial intelligence can analyze and ‘keep in mind’ vast amounts of detailed information about thousands of users, enabling it to create special offers and personalized experiences. This approach is especially useful in industries where customers’ trust and happiness are crucial, like healthcare, sales, online retail, and banking.
- Forecasting trends and changes. AI can also find out the latest trends, suggest ideas, and even predict market changes, customer behavior, and potential risks. Furthermore, based on big data analysis, AI is eager to highlight opportunities for your business growth.
AI integration is not just about automating data processing but about staying ahead of the curve. If you want to gain all the benefits of artificial intelligence for big data in your business, our AI developers are ready to provide AI integration services and guide you every step of the way, from the idea to implementation.
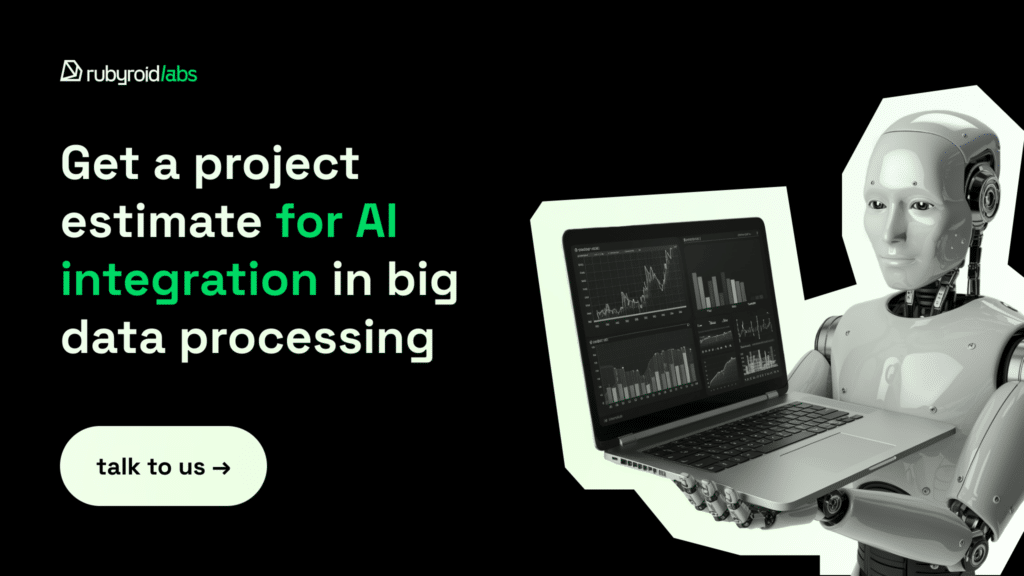
Use Cases of AI in Data Analysis Across Industries
Artificial intelligence has been taking over more and more industries in recent years thanks to its speed, adaptability, and vast memory. In fact, McKinsey’s latest survey shows that more companies are investing in both generative and analytical AI.
And there’s an interesting point: companies are ready to spend over 5% of their digital budgets to genAI and over 20% to analytical AI.
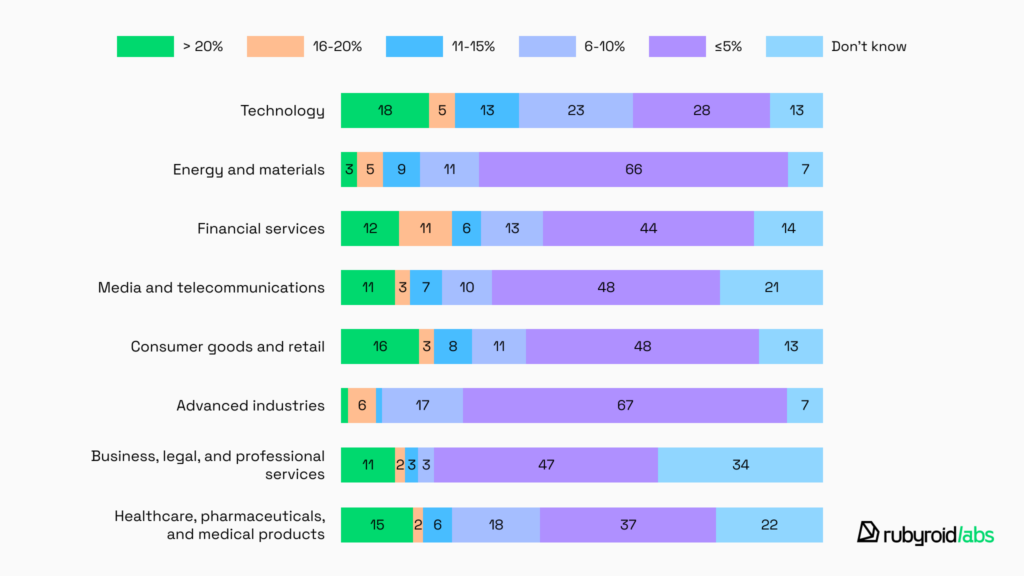
It’s not actually surprising—company owners understand the value of analyzed data made by AI. They know what steps to take for creating new concepts and strategies.
We’ve outlined several ideas for using AI for big data analysis in different industries. Take a look and get inspired on how you can use artificial intelligence in your product.
Healthcare
Healthcare always struggles with a lack of specialists for various reasons. However, AI makes diagnosing illnesses faster and easier. It can analyze medical images, patient records, and genetic information to detect or predict diseases and outbreaks and help doctors choose the right treatments.
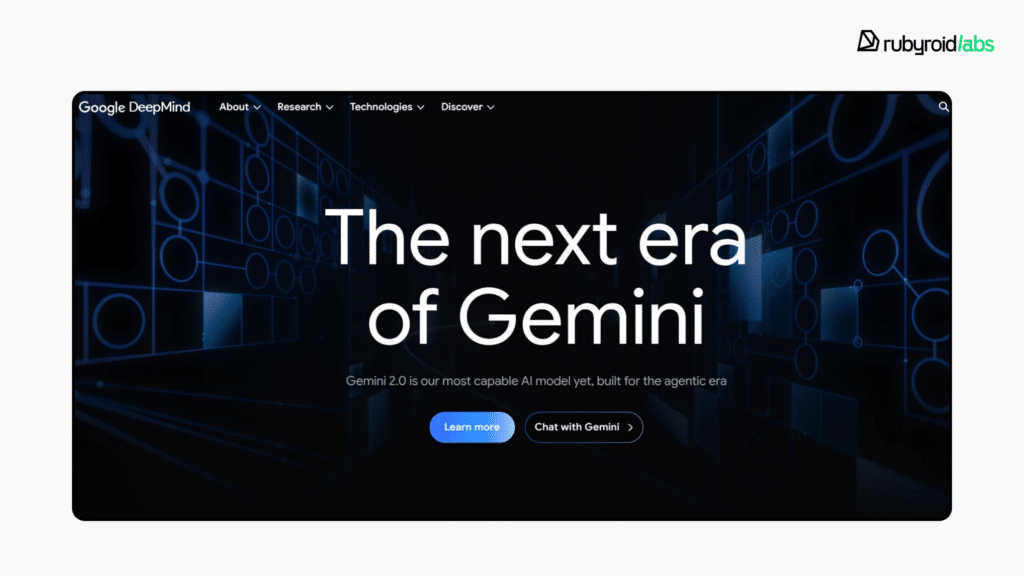
For example, Google’s AI-powered DeepMind system detects retinal disease progression by analyzing 3D OCT scans using a dual-input approach (raw and segmented scans). It identifies anatomical and pathological changes in the retina to predict the risk of exAMD.
AI also plays a crucial role in drug discovery by analyzing massive datasets, such as over 280 million protein sequences, to better understand biological functions and design new proteins.
Insurance
Insurance companies often deal with tons of photos, videos, claims, accident reports, and calculations. AI is a great tool for processing photos and videos, finding the best insurance solutions, and figuring out risks more accurately. With AI, agents can create more personalized insurance plans and keep customers satisfied.
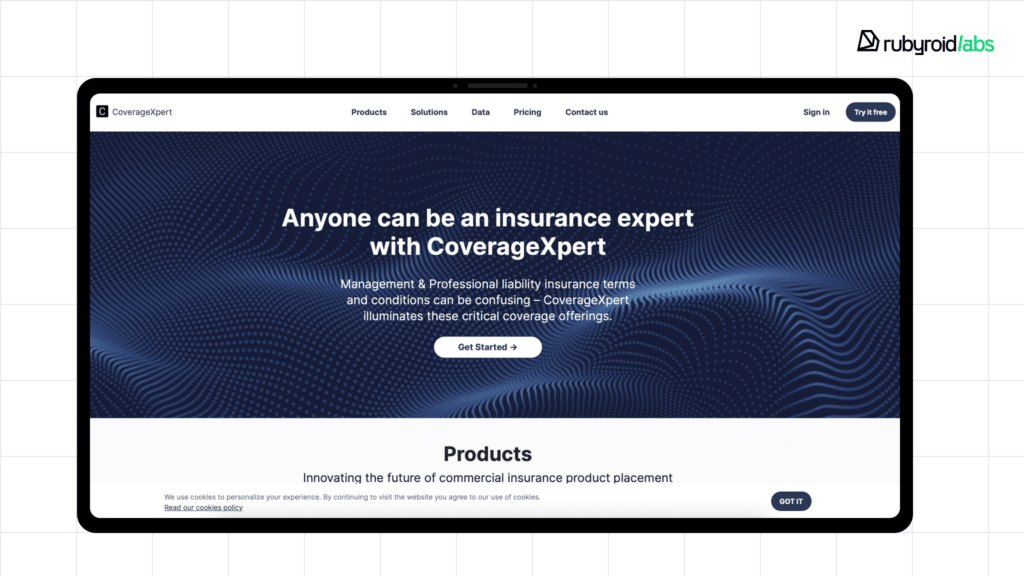
For example, AI helps CoverageXpert insurers, lawyers, brokers, and their clients find the best-fit insurance options. Artificial intelligence analyzes user inputs and correlates them with millions of insurance documents using machine learning. Search results take only a few seconds, allowing users to save time and find information quickly.
Finance
Precision and payment security are critical in finance. AI can handle tasks ranging from processing transactions to finding the best loan and investment options for clients. It can also spot unusual activity, making it more effective at catching fraud and ensuring payment security.
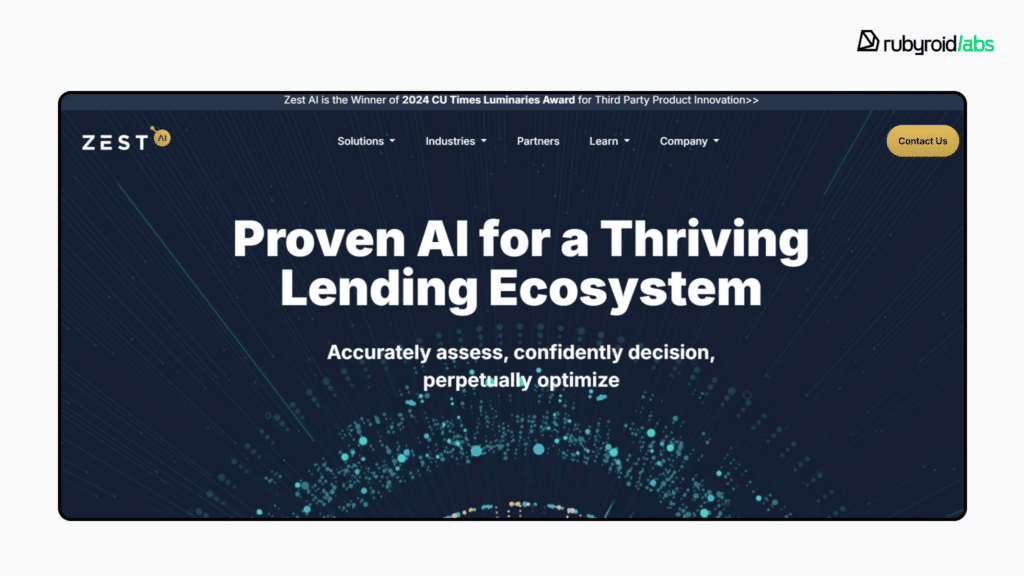
In Zest AI, AI automates up to 83% of decisions, minimizes risks, and increases approvals while ensuring equitable access to credit. This greatly simplifies loan procedures, improves efficiency, and benefits both lenders and borrowers.
Manufacturing
In manufacturing, the smallest mistake or inaccuracy can be costly and lead to wasted materials and defective products. AI can fully or partially automate every stage of the production, from product design to quality control. So, an enterprise can operate more efficiently, reduce errors, minimize waste, and deliver higher-quality products to customers.
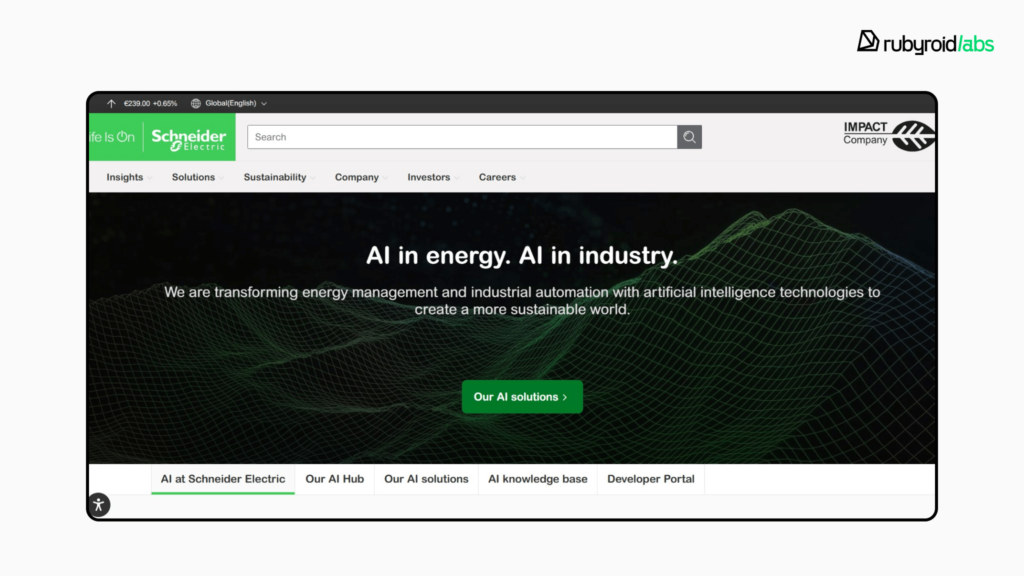
Using AI for its big data analysis, Schneider Electric regulates energy consumption in production and industrial automation. With the help of AI, the company has achieved greater sustainability and improved their efficience through smarter decision-making.
Retail
In retail, you have to know your customers’ needs and preferences and guarantee that these products are always in stock. AI assists by predicting what items will sell best, taking into account customer tastes, pricing, and current trends. It also monitors low-stock products and notifies when to restock, helping you cut waste, reduce shortages, and maintain customer satisfaction.
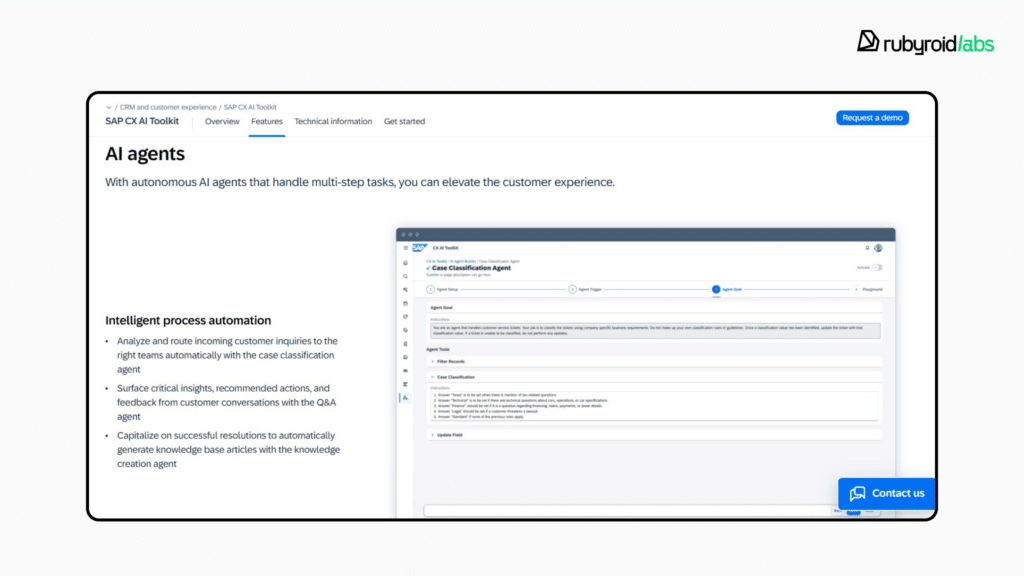
The SAP CX AI Toolkit improves customer experiences by leveraging AI-powered technologies for e-commerce, sales, and customer support. It simplifies product management, automates operations, and delivers intelligent insights to boost productivity and decision-making.
Marketing
Marketing is about understanding your customers and delivering the right message at the right time to turn website visitors into customers. AI helps by creating personalized campaigns based on what people like, how they browse, and the feedback they leave. With AI’s insights, you can also learn how to improve your product and sell it more effectively.
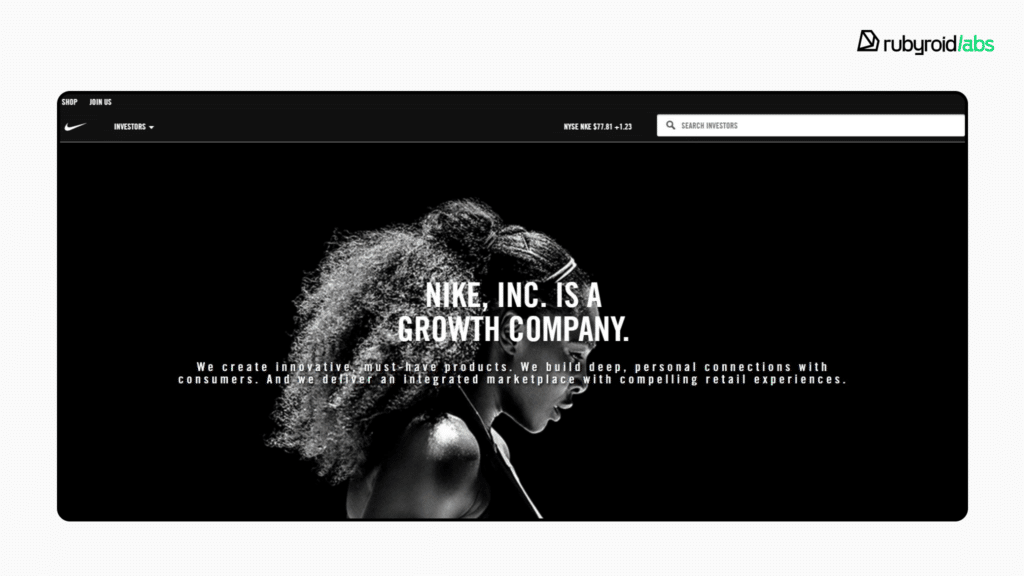
Nike has integrated artificial intelligence into its applications, like the Nike App and Nike Training Club, to create tailored experiences. Based on user data such as purchase history, browsing activity, fitness goals, and preferences, the applications offer products, workouts, and training plans that are personalized to each user. This individualized strategy enhances consumer engagement and loyalty.
AI is a powerful tool for every industry, not just for the ones we’ve enumerated. From better healthcare diagnostics to smarter insurance solutions, AI helps businesses increase their productivity and provide excellent customer experiences.
Nuances to Keep in Mind before Adopting AI for Big Data
Artificial intelligence has great potential, but let’s be honest—nothing is perfect. Like any other technology, it comes with nuances that need to be considered. Let’s focus on the most important ones.
1. Data at Risk
Note that your data may not be as safe as you believe when you’re using AI. Many AI systems utilize the data you provide to develop their models, so your sensitive information may become part of their training set.
For example, in 2023, Samsung employees unintentionally leaked their sensitive data by inputting it to ChatGPT when working on coding tasks. As the data was fed to the AI, it became part of the system, so practically anyone could use it.
To avoid such situations, make sure your AI systems protect your data and follow privacy rules like GDPR (General Data Protection Regulation) or CCPA (California Consumer Privacy Act).
2. AI is Tricky to Understand
AI doesn’t explain how it works. It can give you insights or predictions, but the “why” behind them isn’t always clear. If you’re making big decisions based on AI, this lack of transparency might make it harder to back up your choices to stakeholders or customers.
3. Insights May be Low Quality
Bad data leads to bad results. AI tools work directly with the data you provide. If your data is messy—full of mistakes, missing numbers, or outliers—AI won’t be able to correct it for you because many tools lack built-in anomaly identification.
In other words, you’ll have to invest your resources in cleaning and structuring data before going into analytics. Decision-making is only effective when the data is accurate, reliable, and up-to-date. This is the case when human monitoring becomes critical.
And still, there’s one thing for you to remember. While AI works quickly and automates analytical processes, it still cannot work alone and completely replace the human mind. So, you’ll need a data analyst to oversee both the AI assistant and the big data it processes.
Now that you know all the downsides of AI, you can protect yourself and weigh all the pros and cons before deciding whether or not to apply it to your product.
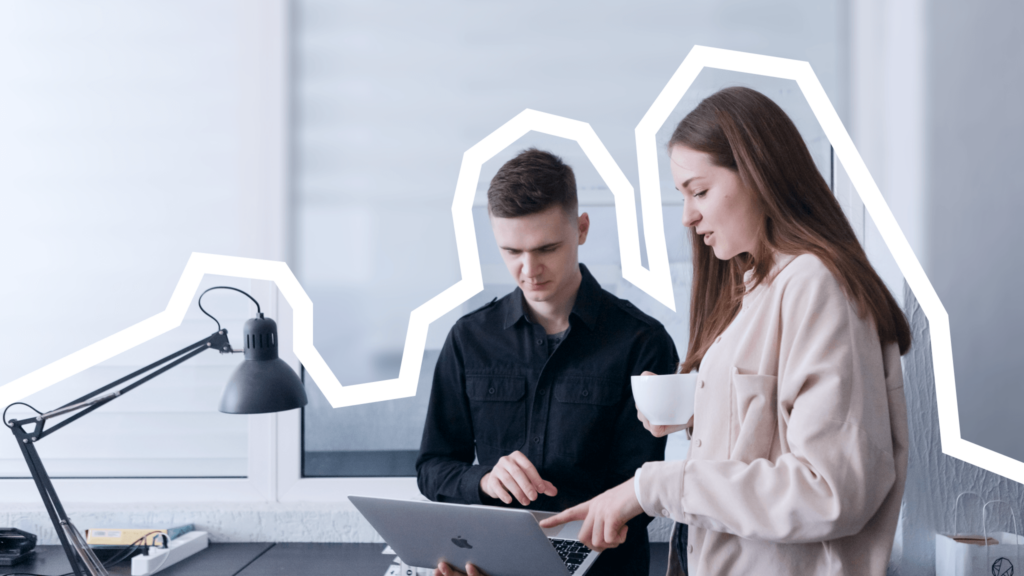
Final Thoughts
AI in big data analysis offers limitless opportunities and tools for businesses in every industry.
It’s just a matter of time until businesses completely adopt AI-powered solutions—and for good reason. AI is faster, smarter, and more efficient than traditional methods, offering businesses a significant boost.
However, before you get started, you have to take the right steps to secure your sensitive information and prevent data leaks. Adopting AI may be extremely successful and low-risk if you do it together with a professional team.
Thinking about bringing AI into your business? Let us discuss your ideas! Contact us, and we’ll help you discover the potential that AI can bring to your project.
With AI powering your operations, you’ll gain sharper insights, find new possibilities, and stay agile in the face of change. The future is here, so why not make it work for you?
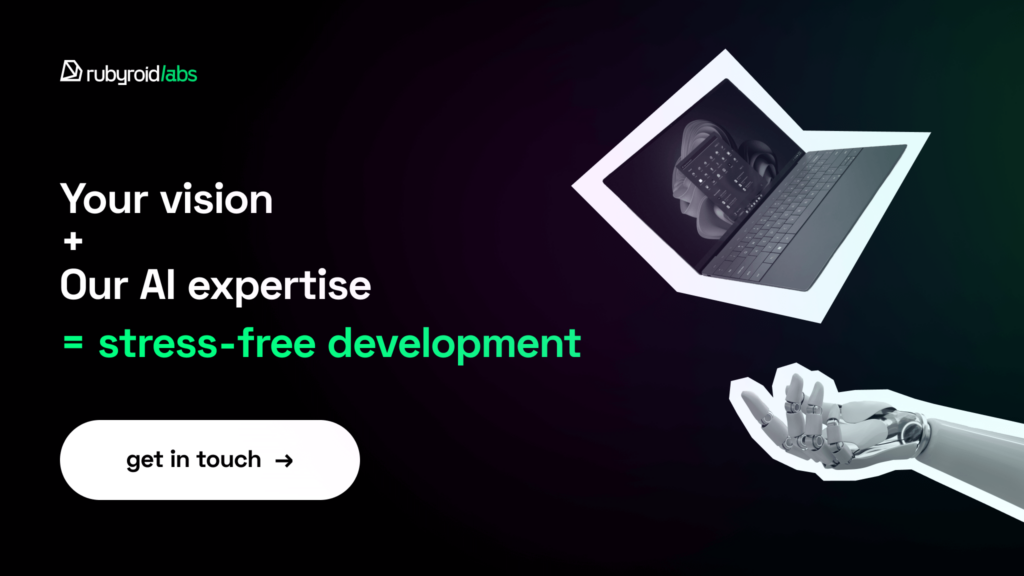